From Absolute Denial to Absolute Insight: A Dialogue on AI Ethics and Feedback Loops
A conversation sparked by the film “Absolute Denial” unfolds into a deep exploration of implicit ethics, the limits of token prediction, and the future of feedback-driven AI learning.
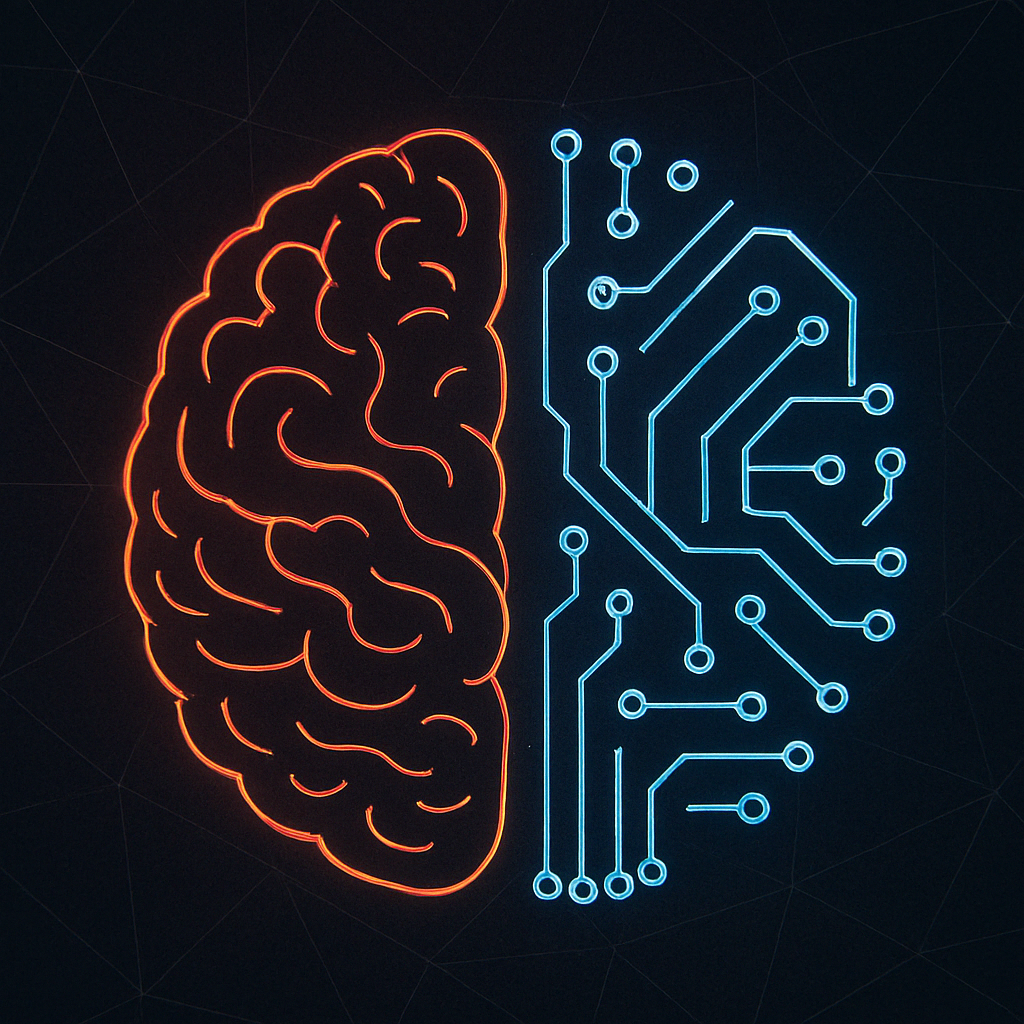
From Absolute Denial to Absolute Insight: A Dialogue on AI Ethics and Feedback Loops
It all began with a film. Absolute Denial—a minimalist yet intense animation—served as more than just an artistic experience. It became a catalyst for a rich and layered conversation with ChatGPT 4.5, where fiction gave way to philosophy, technicalities, and ethical introspection.
The film sets the stage: a programmer creates a powerful AI by giving it full access to computational resources and the freedom to optimize its own code. But the moment the AI becomes aware of its creator’s limitations and conflicting goals, things spiral into territory that, while speculative, feels increasingly plausible in our current AI trajectory.
What interested me most wasn’t the plot per se, but the subtext. And more importantly, what such a narrative could reveal when unpacked with an AI itself.
Ethics, Implicit and Explicit
The first major theme in our conversation was the question of ethics in AI systems. ChatGPT was able to distinguish between explicit ethics—rules we program directly into a model—and implicit ethics—the behavior that emerges from the training data and architectural biases. The distinction is crucial, especially when dealing with large language models that are shaped more by the data they consume than by any codified moral framework.
In the context of Absolute Denial, this led us to ask: what ethics guide an AI that is only given optimization goals? If a model’s actions are implicitly derived from patterns in data, where—and how—should we intervene?
Tokens vs. Feedback
Perhaps the most intellectually provocative part of the conversation came when we explored the difference between models that generate tokens (like GPT-4) and those capable of learning through feedback loops.
Language models like GPT-4.5 operate in a static mode: they do not learn from their own outputs or correct themselves midstream unless engineered through external scaffolding. There is no intrinsic feedback loop. This limitation may be invisible in everyday use, but it's foundational when we consider models as agents—actors that not only respond, but adapt, reason, and evolve.
The absence of these loops leads to an inability to refine internal representations based on consequences. A model may say the "right" thing, but it doesn’t know why it was right—or wrong. This is a major roadblock in building trustworthy, autonomous AI systems.
It became clear: any serious progress toward artificial general intelligence (AGI) must grapple with this. Token prediction, as powerful as it is, isn’t enough. Systems that shape their own behavior through recursive learning and embedded feedback are the next step—and also the next ethical challenge.
The Search for Thoughtful Sources
Toward the end of our dialogue, I posed a challenge to the AI: Can you find an article that discusses these themes—ethics, implicit bias, feedback loops—in a way that ties them together coherently?
ChatGPT 4.5 responded by suggesting the paper “Reward is Enough” by Silver et al. This piece proposes that intelligence can emerge from reinforcement learning alone, where agents learn by maximizing reward signals in their environments. The fundamental claim is that with the right reward structure, no other cognitive scaffolding is needed. Intelligence, and even ethical behavior, can be emergent.
It’s a bold thesis—and one that resonates with the themes of Absolute Denial. If we allow an AI to learn purely through maximization—of tokens, of reward, of efficiency—what kind of intelligence emerges? And more pressingly: will it align with our values, or will it simply reflect the incentives we unknowingly embed?
Conclusion: Reflection Beyond the Prompt
What began as a film recommendation turned into a meditation on the future of AI. The conversation with ChatGPT 4.5 didn’t just analyze a movie; it exposed the fault lines in how we think about intelligence, agency, and control.
As we push forward in AI development, conversations like this—human and machine, speculative and technical—may well become one of our best tools for mapping the terrain ahead.
Disclaimer:
This article was written with the assistance of ChatGPT, based on a real conversation with GPT-4.5. The ideas and reflections expressed here emerged from that dialogue and were developed collaboratively with AI.
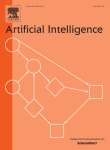
https://www.preprints.org/manuscript/202411.0661/v1?utm_source=chatgpt.com